THE PRACTICAL GUIDE TO DATA MESH
Setting up and supervising an enterprise-wide data mesh
Overview
Data Quality usually refers to a company’s ability to ensure the longevity of its data. At Zeenea (a data catalog provider), we believe Data Quality is ensured through the 9 following dimensions - all essential to extract value to your company:
🔸 Completeness
🔸 Accuracy
🔸 Validity
🔸 Uniqueness
🔸 Consistency
🔸 Timeliness
🔸 Traceability
🔸 Clarity
🔸 Availability
We will detail these dimensions with the help of a simple example in part one. We will then elaborate on how Data Quality management is an important challenge for organizations seeking to extract maximum value from their data.
We will also draw parallels between these different Data Quality dimensions and the different risk management phases to overcome - identification, analysis, evaluation, and processing. This will enable you to hone your risk management reflexes by tying in Data Quality improvement processing to a company objective (and evaluating the ROI on each quality dimension).
Once we have established the main features of an enterprise Data Quality management tool, we will detail how a Data Catalog - though not a Data Quality tool - can contribute towards Data Quality improvement (through the clarity, availability, and traceability dimensions mentioned above).
Ebook overview
Centralized and monolithic data management, anchored in a data lake or warehouse, creates a massive bottleneck that stifles innovation and hinders data teams from meeting the rapidly evolving demands of the business. In response, the industry is progressively embracing decentralized data management, especially through the data mesh.
Introduced by Zhamak Dehghani in 2019 and inspired by Amazon's early 2000s transformation, this paradigm shift is grounded in four fundamental principles: domain-oriented decentralized data ownership and architecture, data as a product, self-serve data infrastructure as a platform, and federated computational governance.
While the data mesh is well-documented, literature often portrays an idealized end state without detailing the practical steps to achieve it. This gap raises a crucial question: how can organizations effectively transform their data management practices and implement a data mesh?
« In this eBook, I offer a detailed iterative approach to building a data mesh, rooted in its four core principles and enriched by lean manufacturing methodologies. This approach is based on the idea that building must be viewed as a learning process, one that organizations can embark on swiftly by leveraging their existing human and technological resources. »
— Guillaume Bodet, Co-founder & CPTO, Zeenea
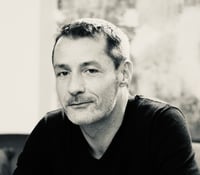
This guide is designed to arm you with practical strategies for implementing data mesh in your organization, helping you:
✅ Understand the critical factors behind the rise of decentralized data management and data mesh adoption
✅ Start your data mesh journey with a focused pilot project, leveraging an initial use case
✅ Discover efficient methods for scaling up your data mesh, enhancing the creation of data products
✅ Acknowledge the pivotal role an internal marketplace plays in facilitating the effective consumption of data products
✅ Learn how Zeenea emerges as a robust supervision system, orchestrating an enterprise-wide data mesh